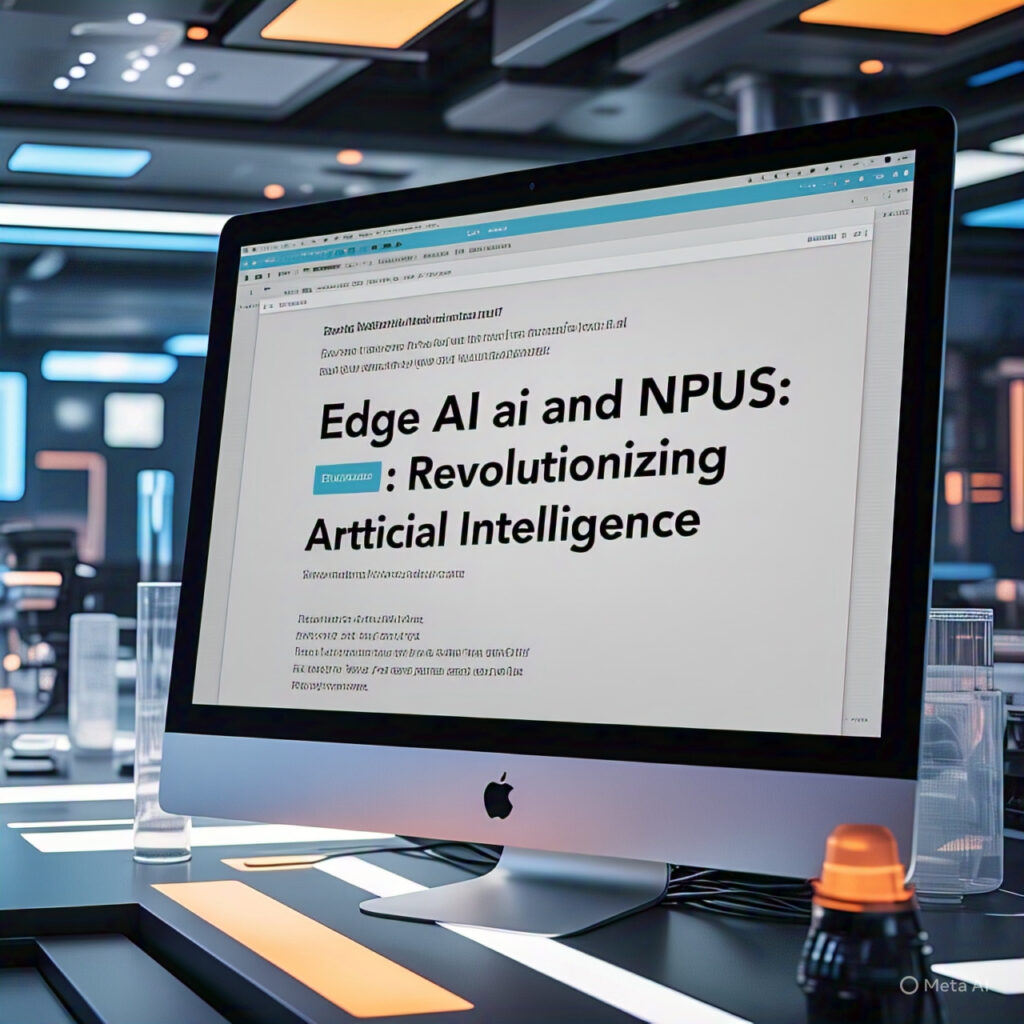
Edge AI Technology represents a transformative shift in the deployment of artificial intelligence,
whereby data is processed at or near the source rather than relying on centralized cloud-based solutions.
This approach provides several advantages, notably reduced latency, improved bandwidth efficiency, and enhanced privacy.
By bringing intelligence closer to the data generation point, Edge AI reduces the need for extensive data transfers, which can lead to bottlenecks and delays in decision-making processes.
This technology is particularly beneficial for applications that require real-time processing, such as autonomous vehicles, smart manufacturing systems, and various Internet of Things (IoT) devices.
Integral to the function of Edge AI are Neural Processing Units (NPUs), specialized microprocessors designed to efficiently execute machine learning algorithms.
NPUs are optimized specifically for neural network computations, enabling them to perform complex AI tasks more quickly and with less energy than traditional processors.
By enhancing computational capabilities at the edge, these units enable devices to analyze, interpret, and respond to data in real-time, significantly augmenting their functionality.
The ability of NPUs to manage vast amounts of data with minimal power consumption makes them an attractive option for myriad applications, particularly in environments where energy efficiency is paramount.
This shift towards a more decentralized computational architecture not only enhances performance but also enables new business models across various industries.
For instance, in healthcare, Edge AI can facilitate immediate patient monitoring, providing rapid diagnostics while safeguarding sensitive information.
In retail, it can streamline inventory management by enabling instantaneous data analysis.
As industries increasingly adopt these advancements, the importance of Edge AI and NPUs becomes clear, signifying a pivotal movement towards smarter, more responsive technology systems.
Videos will be added as random thoughts to Edge AI technology…
Microprocessors
The technological landscape of computing has witnessed dramatic transformations since the inception of microprocessors in the early 1970s.
The first microprocessor, the Intel 4004, marked a pivotal moment by integrating all the components of a computer’s central processing unit (CPU) onto a single chip.
This innovation laid the groundwork for subsequent developments that would eventually enable powerful computations in compact forms.
Over the decades, microprocessors have evolved significantly, becoming faster and more efficient due to continuous advancements in semiconductor technology.
The 1980s and 1990s saw the introduction of microprocessors with diverse architectures, aiming to enhance performance while navigating limitations.
Technology advancements, such as Moore’s Law, facilitated the miniaturization of transistors.
This principle predicts that the number of transistors on a microchip doubles approximately every two years, resulting in increased performance and reduced costs for consumers.
As a result, microprocessors transitioned from 8-bit and 16-bit architectures to more complex 32-bit and later 64-bit designs, accommodating increasingly sophisticated applications.
As computational demands surged with the advent of the internet and mobile devices,
microprocessor developers began prioritizing power efficiency alongside performance.
This dual focus led to the introduction of multi-core processors, allowing manufacturers to achieve higher throughput without proportionally increasing power consumption.
Such advancements proved crucial for applications requiring robust processing capability, such as artificial intelligence (AI).
In recent years, the emergence of integrated neural processing units (NPUs) represents a significant milestone in microprocessor evolution.
Designed specifically to handle AI tasks efficiently, NPUs leverage the existing computational power of microprocessors while enhancing their ability to process vast amounts of data.
This integration optimizes performance for AI workloads while minimizing latency, thereby shaping the future of edge computing and AI-driven applications.
How NPUs Operate
Neural Processing Units (NPUs) have emerged as a critical component in the landscape of artificial intelligence, particularly in edge computing environments.
The architecture of NPUs is specifically designed to optimize the execution of neural networks,
enabling advanced machine learning tasks to be performed efficiently and effectively.
Unlike traditional CPUs or GPUs, NPUs utilize a specialized architecture that facilitates parallel processing,
which is essential for handling the massive amounts of data typical in AI applications.
At the core of an NPU’s architecture are multiple processing cores that can operate simultaneously.
This parallelism allows for the rapid completion of complex computations involved in training and running neural networks.
The NPU architecture often incorporates dedicated hardware blocks for common operations such as matrix multiplication and convolution,
which are fundamental to deep learning algorithms.
This dedicated processing capability means NPUs can execute these tasks at significantly higher speeds compared to general-purpose processors,
reducing latency and energy consumption.
Data flow management is another key aspect of how NPUs operate.
To optimize the efficiency of computations, NPUs employ data pipelining techniques that allow for the overlapping of data fetch operations with computation processes.
By minimizing bottlenecks, NPUs can ensure that cores remain active and are not idly waiting for data to be available, which is a common issue in traditional processing architectures.
Moreover, the use of specialized algorithms is vital for enhancing the performance of NPUs.
These algorithms are typically tailored to leverage the unique architecture of NPUs,
allowing for faster and more efficient learning processes.
For instance, algorithms designed for quantization can help in reducing the precision of data without significantly impacting the accuracy of predictions,
thus enabling NPUs to work effectively even with limited resources.
The Benefits of Edge AI with Integrated NPUs
Deploying AI at the edge with integrated Neural Processing Units (NPUs) presents a multitude of benefits that contribute to the overall efficiency and effectiveness of data processing.
One of the primary advantages of edge AI is the significantly improved response times.
By processing data locally, edge devices can analyze information in real-time, leading to immediate responses and decisions.
This is particularly crucial in applications such as autonomous vehicles, industrial automation, and smart homes, where timely actions can enhance safety and operational efficiency.
Enhanced privacy and security is another notable benefit of integrating NPUs on edge devices.
Traditional AI solutions rely heavily on cloud infrastructure, wherein sensitive data is transmitted over the internet for processing.
This can expose user information to potential breaches or unauthorized access.
However, by leveraging NPUs at the edge, data can be processed locally, ensuring that personal and sensitive information remains within the device.
This reduces the risk of data leaks and fosters greater trust among consumers regarding their privacy.
Furthermore, the use of integrated NPUs lessens the reliance on cloud infrastructure.
Many organizations face challenges related to bandwidth limitations and latency issues when relying on cloud-based AI services.
With edge AI, data can be processed swiftly on-site, alleviating these concerns and allowing for continuous operations even in instances of poor network connectivity.
This decentralized approach is not only advantageous for real-time applications but also contributes to a more resilient and reliable system overall.
Lastly, energy efficiency is significantly improved with the deployment of NPUs at the edge.
Edge devices typically consume less power compared to traditional cloud-based systems due to reduced energy-intensive data transfers.
By performing computations locally, these devices can optimize their energy usage, ultimately leading to cost savings and a reduced carbon footprint.
Applications of Edge AI and NPUs
The proliferation of Edge AI, particularly through the integration of Neural Processing Units (NPUs),
has led to transformative advancements across several sectors, notably in
- healthcare,
- automotive,
- smart cities,
- and Internet of Things (IoT) devices.
These applications capitalize on the ability of localized data processing, enabling swift decision-making and enhanced efficacy.
In healthcare, the use of Edge AI is making significant strides.
Medical devices equipped with NPUs can perform real-time analysis of patient data, leading to timely diagnostics and personalized treatment plans.
For instance, wearable devices monitoring heart rates and other vital signs can alert both patients and doctors of any anomalies immediately,
facilitating proactive medical interventions.
Edge AI technology
This capability not only improves patient outcomes but also alleviates the burden on healthcare systems by preventing more severe health crises.
In the automotive sector, particularly in the realm of autonomous vehicles, NPUs enhance safety and driving efficiency.
With the ability to process vast amounts of data from on-board sensors and cameras in real-time,
vehicles can make informed decisions
—whether navigating through traffic or avoiding obstacles.
Companies like Tesla and Waymo utilize integrated NPUs to develop sophisticated algorithms that predict and react to environmental changes instantaneously, fostering safer roads and more reliable transport systems.
Smart cities are another area reaping the benefits of Edge AI Technology.
Through intelligent traffic management systems equipped with NPUs,
cities can analyze patterns and optimize traffic flows,
thereby reducing congestion and lowering pollution levels.
Moreover, smart surveillance systems, utilizing Edge AI technology, enhance public safety by providing real-time monitoring and threat detection without the latency prevalent in traditional cloud-based systems.
Ultimately, the rise of Edge AI and NPUs is shaping a future where efficiency, safety,
and enhanced user experiences are pivotal,
highlighting a trend that is likely to expand further across various sectors,
once again proving the transformative potential of technology in modern society.
Challenges and Limitations of Current Technologies
As microprocessors evolve to incorporate integrated Neural Processing Units (NPUs), several challenges and limitations hinder their widespread adoption.
One significant issue arises from the lack of standardization across varying platforms, which complicates the development and deployment of applications using NPUs.
Different vendors may implement neural architecture differently, leading to compatibility issues that could stifle innovation and limit the efficiency of applications relying on AI functionalities.
This variety can deter developers who are hesitant to invest time and resources into hardware that may not work seamlessly across devices or systems.
Another notable challenge lies in the inherent complexity of programming for heterogeneous hardware.
NPUs are typically integrated with CPUs and GPUs, which possess distinct architectures and processing methodologies.
This complexity can lead to difficulties in optimizing performance, as developers must navigate a convoluted environment where tasks need to be efficiently distributed across different processing units.
The learning curve associated with mastering these diverse systems adds to the time and financial investments required for application development, potentially stalling progress in the field.
Power consumption presents yet another challenge.
As AI workloads increase in size and complexity, so too do their energy demands.
The design of NPUs strives to balance performance with power efficiency; however, achieving this equilibrium remains a daunting task.
Excessive power usage not only raises operational costs but may also lead to thermal management issues, affecting the stability and reliability of devices.
Furthermore, budget constraints could limit the advancement of technology, as enhanced capabilities often come at a higher price,
prompting organizations to weigh cost against performance.
Addressing these challenges is crucial for realizing the full potential of integrated NPUs in the realm of microprocessing.
Future Trends in Microprocessors with Integrated NPUs
The evolution of microprocessors with integrated Neural Processing Units (NPUs) is expected to significantly influence the edge AI technology landscape in the coming years.
A notable trend is the advancement in artificial intelligence (AI) algorithms, which are becoming increasingly sophisticated,
allowing for more efficient processing of tasks that require machine learning and deep learning capabilities.
These improved algorithms enable microprocessors to perform complex computations with reduced energy consumption and latency, making them ideal for edge computing applications.
Another emerging trend is the rise of new programming paradigms specifically designed to optimize the performance of NPUs.
These paradigms prioritize parallel processing and take advantage of specialized hardware architectures, leading to enhanced computational efficiency.
As developers increasingly adopt these new methodologies, it is anticipated that software will become more tightly integrated with hardware,
resulting in systems that can adapt to user needs in real-time while maintaining high performance standards.
Sustainability in hardware design is also gaining traction, with manufacturers focusing on producing energy-efficient microprocessors with integrated NPUs.
This emphasis on eco-friendly computing solutions reflects a growing awareness of the environmental impact of technology.
Efforts to reduce the carbon footprint of microprocessors include using sustainable materials and designing chips that operate at lower power levels without sacrificing performance.
Innovations in fabrication techniques, such as advancements in semiconductor materials and processes, are expected to play a crucial role in this transition.
As these trends continue to develop,
the future of microprocessors equipped with NPUs appears promising.
Innovations in AI, programming methods, and sustainable practices are likely to create opportunities for enhanced performance,
greater accessibility, and lower environmental impact.
This alignment of technological advancements and societal values may well shape the next decade of computing technology.
The Role of Leading Tech Companies
The advancement of microprocessors with integrated Neural Processing Units (NPUs) has been significantly influenced by major technology firms in the industry.
These companies are at the forefront of research and development (R&D) in artificial intelligence (AI) and edge computing,
driving innovations that enhance the functionality and efficiency of AI applications.
By leveraging their expertise, leading tech firms are contributing to a competitive landscape that fosters rapid progress in the deployment of NPUs.
For instance, companies like NVIDIA and Intel have made substantial investments in developing microprocessors that incorporate NPUs,
focusing on optimizing performance for AI workloads.
NVIDIA, with its renowned GPU technology, has expanded its product line to include processors specifically designed for edge AI applications.
This strategic move underscores the company’s commitment to improving computational capabilities and energy efficiency at the edge.
Similarly, Intel is advancing its Intel Nervana architecture,
which is tailored for deep learning tasks,
thereby solidifying its positioning in the market.
Moreover, partnerships play a crucial role in the proliferation of microprocessors with integrated NPUs.
Tech giants are forming alliances with smaller startups and research institutions to enhance their technological capabilities.
For example, collaboration between established firms and agile startups allows for the sharing of expertise and resources,
which accelerates innovation in neural processing.
This synergy between large corporations and nimble players fosters a dynamic ecosystem that cultivates breakthroughs in AI-processing technology.
In addition to R&D and partnerships, these companies are also strategically positioning themselves in various market segments to capture emerging opportunities associated with microprocessor technology.
Conducting extensive market research, they aim to understand customer needs and tailor their products accordingly.
As competition intensifies, the focus on developing diverse applications for microprocessors with NPUs is likely to result in significant advancements in AI capabilities across industries.
Conclusion
As we navigate the advancements in technology,
it becomes increasingly clear that the rise of microprocessors with integrated Neural Processing Units (NPUs) is revolutionizing the way we interact with artificial intelligence (AI).
This shift, known as Edge AI technology, brings the computational power of machine learning directly to devices,
enabling real-time processing without relying on cloud-based servers.
This transformation is not only reshaping industrial standards but also significantly impacting everyday consumers and businesses.
Edge AI empowers smart devices, allowing them to process information locally, which enhances performance while minimizing latency.
For consumers, this means faster and more responsive gadgets—from smartphones that understand context to home assistants capable of executing tasks with minimal delay.
The seamless operation of devices around us is a testament to the capabilities that NPUs offer, effectively integrating intelligent functionalities into our daily lives.
For businesses, the implications are profound.
Organizations are increasingly leveraging Edge AI technology solutions to optimize operations,
enhance customer experiences, and improve decision-making.
The deployment of NPUs in industrial applications allows for smarter manufacturing,
predictive maintenance, and real-time data analytics,
which are crucial for maintaining competitiveness in a rapidly changing market.
Companies that adopt Edge AI technologies position themselves at the forefront of innovation,
ready to capitalize on efficiencies that were previously unattainable.
Embracing this Edge AI revolution is not merely about adopting new technologies;
it involves a cultural shift towards understanding and applying intelligence in a decentralized manner.
The integration of NPUs into everyday devices is fostering a future where AI transcends limitations,
allowing both consumers and enterprises to harness its full potential.
The blessings of this transformative wave are ever-greater
- accessibility,
- efficiency,
- and connectivity,
making the case for the urgent need to embrace Edge AI all the more compelling.
Thanks – Let me know of your thoughts 💭💭…